Insights from My Journey of Launching AI Products
- kulkarnisachind2
- Sep 2, 2024
- 4 min read
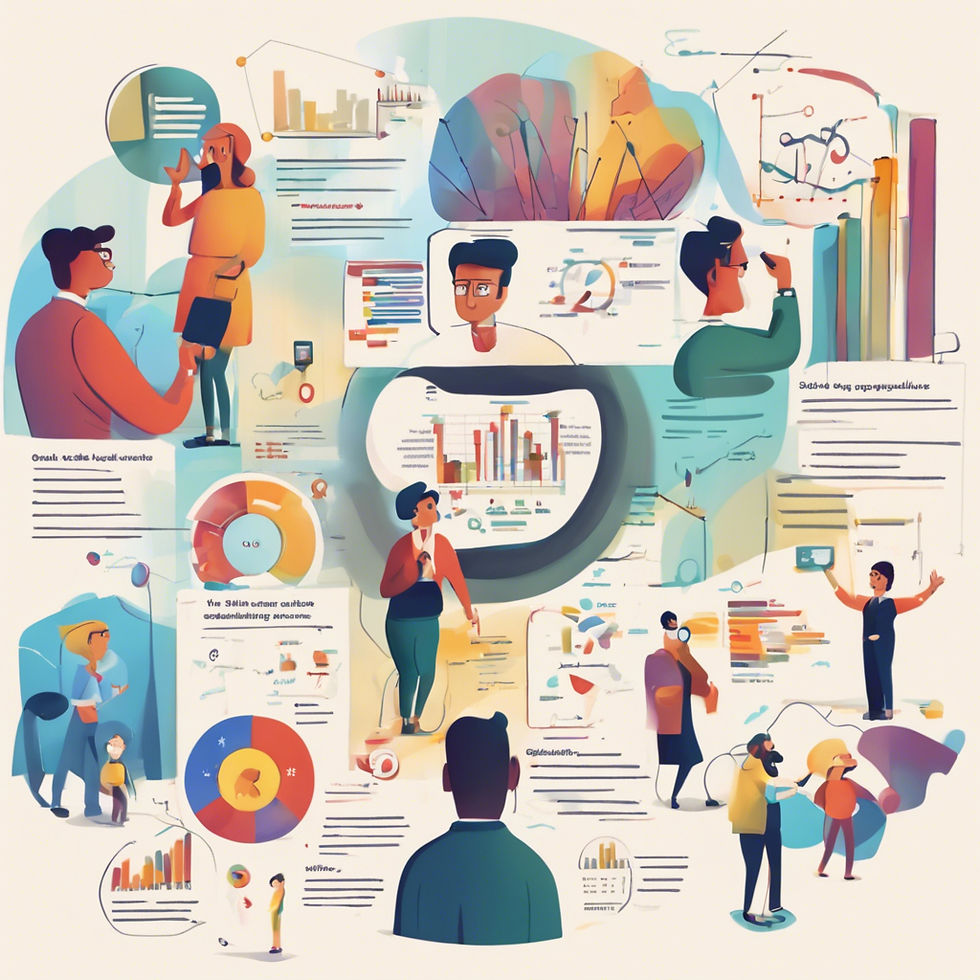
Sachin's Take - With extensive experience in launching numerous AI products and platforms, I've identified the five critical components that guarantee a successful launch:
High-quality Data
Skilled Team
Effective Model
Clear Goal
Well-defined Problem
My experience with AI Products
I have extensive hands-on experience in ideating and launching AI platforms like
Predicted gross bookings (pGB)
Lifetime value (LTV)
Message personalization
Omni-channel message orchestration across 8 channels
Content optimization
Predictive user segmentation
My Learnings
The five top learnings after building multiple AI products are:
Data is Paramount: Treat data as the foundation of your AI product's success.
Collaborate with Top Talent: Engage the best applied and data scientists to drive innovation.
Clearly Define Goals: Establish clear and measurable objectives from the outset.
Begin with a Model and Iterate: Start with a baseline model and continuously refine it.
Understand Real-World Challenges: Be aware of the practical challenges in building and deploying AI products.
#1 Data is Paramount
Quality and Relevance: Ensure that the data you collect is of high quality and relevant to the problem you're trying to solve. Garbage in, garbage out is particularly true in AI.
Data Privacy and Security: Safeguard your data by implementing robust privacy and security measures, complying with regulations such as GDPR or CCPA.
Volume and Diversity: Gather a large and diverse dataset to improve the generalizability and robustness of your AI models. Diverse data helps in avoiding biases and ensures better performance across different scenarios.
Data Annotation and Labelling: Properly annotate and label your data. Accurate labelling is crucial for supervised learning models and significantly impacts the model's performance.
Continuous Data Updates: Establish a process for continuously updating your dataset. AI models need to evolve with changing data patterns to remain effective and accurate.
#2 Collaborate with Top Talent
Expertise and Experience: Assemble a team of experts with experience in AI, data science, and relevant domain knowledge. The quality of your product is directly linked to the skills of your team.
Interdisciplinary Collaboration: Encourage collaboration between data scientists, engineers, domain experts, and business stakeholders to ensure all perspectives are considered in the product development.
Training and Development: Invest in ongoing training and development for your team. AI is a rapidly evolving field, and staying up-to-date with the latest advancements is essential.
Diverse Perspectives: Build a diverse team to bring different viewpoints and ideas to the table, which can lead to more innovative solutions and help mitigate biases.
Communication Skills: Ensure team members have strong communication skills to effectively translate technical findings into actionable insights for non-technical stakeholders.
#3 Clearly Define Goals
Clear Objectives: Define clear, measurable objectives for your AI product. Know what problem you are solving and what success looks like.
Alignment with Business Strategy: Ensure that your AI goals align with the broader business strategy and objectives. This alignment helps in securing support and resources from stakeholders.
Customer-Centric Focus: Set goals that prioritize customer needs and provide real value. Understanding the customer's pain points and addressing them should be at the core of your goals.
Scalability and Sustainability: Design goals with scalability and long-term sustainability in mind. Ensure the AI product can grow and adapt over time.
Ethical Considerations: Incorporate ethical considerations into your goals. Ensure your AI solutions are fair, transparent, and do not perpetuate biases or harm.
#4 Begin with a Model and Iterate
Model Selection: Choose the appropriate model type based on the problem and data. Different problems require different approaches, from supervised learning to reinforcement learning.
Model Training: Train your models thoroughly using the collected data, and validate them with separate test sets to ensure they generalize well to new data.
Model Evaluation: Regularly evaluate your models using relevant metrics to assess their performance. Accuracy, precision, recall, and F1 score are common metrics depending on the use case.
Iterative Improvement: Continuously refine and improve your models. AI development is iterative, and regular updates and retraining are necessary to maintain performance.
Deployment and Monitoring: After deployment, monitor the model’s performance in the real world. Be prepared to make adjustments based on feedback and changing data patterns.
#5 Understand Real-World Challenges
Theoretical Foundation: Ensure a strong theoretical foundation for your AI models. Understanding the underlying principles helps in building robust and effective solutions.
Practical Implementation: Balance theory with practical implementation. Develop practical skills to implement theoretical concepts effectively in real-world applications.
Prototyping: Create prototypes to test and validate theoretical models in practical settings. This helps identify potential issues early and allows for adjustments.
Real-World Constraints: Consider real-world constraints such as computational resources, data availability, and operational costs while building AI solutions.
Feedback Loop: Establish a feedback loop between theory and practice. Use practical insights to refine theoretical models and ensure they are applicable and effective in real-world scenarios.
If you are interesting in collaborating with me, contact now!
Comments